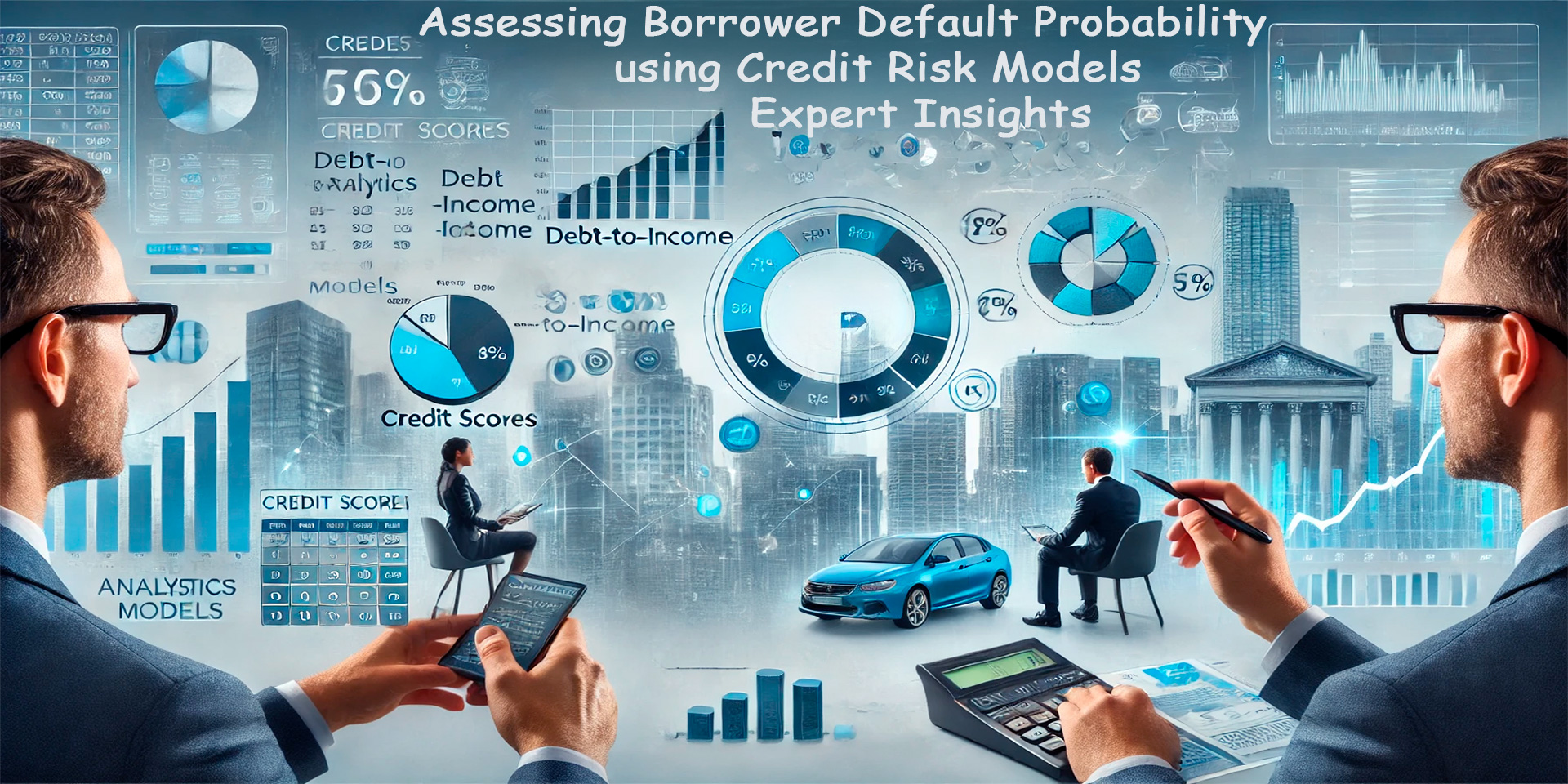
Assessing Borrower Default Probability using Credit Risk Models: Expert Insights
Credit risk models help by analyzing a variety of factors to predict if a borrower might default on their loans. These models use historical data, advanced statistics, and sometimes even machine learning to make accurate predictions. By including elements like credit scores, financial ratios, and economic conditions, they provide a comprehensive view of a borrower’s risk profile.
Machine learning takes this a step further by processing massive amounts of data to identify patterns that might not be evident through traditional methods. Algorithms like random forests and XGBoost can sift through credit histories and loan details to offer precise default probabilities. This data-driven approach means lenders can tailor their loan terms, interest rates, and risk management strategies more effectively, minimizing defaults and optimizing returns.
For instance, a company might call you to let you know how incorporating borrower relationships into credit risk models can enhance accuracy. By adding data like transaction histories and customer interactions, lenders can better gauge a borrower’s reliability. This not only helps in predicting risk but also in creating personalized credit products, thereby increasing customer satisfaction and reducing the likelihood of defaults. Overall, understanding and leveraging default probability models can significantly aid in making smarter lending decisions.
What Are The Key Factors That Influence A Borrower's Default Probability?
Several key factors influence a borrower's default probability:
Credit Score
First, your credit score plays a crucial role. A high credit score indicates reliable debt repayment behavior, reducing your default risk, while a low score suggests missed payments and a higher risk of default.
Debt-to-Income Ratio (DTI)
Next, consider your debt-to-income ratio (DTI). This ratio compares your total monthly debt payments to your gross monthly income. A high DTI means you have significant debt relative to your income, increasing your likelihood of default.
Loan-to-Value Ratio (LTV)
Another important factor is the loan-to-value ratio (LTV). This ratio, which compares the loan amount to the appraised value of the collateral, indicates risk. Higher LTVs mean you have a larger loan compared to the collateral value, increasing default risk.
Employment Status
Your employment status also matters. Stable employment suggests consistent income, lowering default risk. Frequent job changes or unemployment can increase your default probability.
Loan-Specific Factors
- Larger loan amounts carry higher default risk due to bigger repayment obligations.
- Higher interest rates increase the cost of debt servicing, raising default risk.
- Longer loan terms heighten default risk as your financial situation might change over time.
- Loans with collateral generally have lower default risk since they provide security for the lender.
Economic Conditions
Economic conditions, such as unemployment rates, GDP growth, and inflation, affect your financial stability, with adverse conditions increasing default risk. Additionally, the performance of your industry can impact your ability to repay loans. Poor industry health raises default risk.
Financial Stability
Finally, your overall financial stability, including your assets and liabilities, influences your default probability. Stable finances lower your risk, while instability does the opposite.
Finally, understanding these factors—credit score, DTI, LTV, employment status, loan specifics, economic conditions, industry performance, and financial stability—can help you gauge your default risk and make informed lending decisions.
How Do Credit Risk Models Help In Assessing Borrower Default Probability?
Credit risk models help you assess borrower default probability by analyzing various factors to predict whether you will fail to meet debt obligations. These models use historical data, statistical techniques, and machine learning algorithms to estimate the likelihood of default.
Credit scoring models, such as the FICO score, use historical data and statistical techniques to assign a credit score, reflecting your creditworthiness. Default probability models estimate your likelihood of default by analyzing financial indicators, market conditions, and personal characteristics. Techniques include logistic regression and survival analysis. Machine learning algorithms like Random Forest, Support Vector Machines, and Decision Trees analyze large datasets to identify patterns related to your creditworthiness and predict the probability of default.
By using these models, lenders can:
- Evaluate your ability to repay loans.
- Make informed decisions on lending terms.
- Mitigate potential losses and manage risk effectively.
In closing, credit risk models provide precise, actionable insights that help lenders assess your risk level and make sound financial decisions.
What Is The Role Of Machine Learning In Predicting Borrower Default Probability?
Machine learning plays a crucial role in predicting borrower default probability by using advanced algorithms to analyze and interpret vast amounts of data. Here’s how it works:
- Data Collection and Preprocessing: Lenders gather historical loan data and other relevant borrower information. You ensure this data is cleaned and consistent for quality results.
- Feature Selection: Next, you identify key features like credit history and loan amount through feature selection. Techniques like Weight of Evidence encoding help preprocess these variables, making them suitable for model training.
- Model Training: Then, you train various machine learning algorithms such as Random Forest, XGBoost, and LightGBM on the processed data. These models detect complex patterns and relationships not easily seen through traditional methods.
- Prediction and Evaluation: Once trained, your models predict default risk for new borrowers. You evaluate their performance using metrics like accuracy, precision, and AUC. Modern models like XGBoost and LightGBM often provide high predictive accuracy.
- Decision Making: Finally, you use these predictions to make informed decisions about loan approvals, interest rates, and risk management. This helps minimize defaults and optimize financial returns.
Overall, machine learning gives you a reliable and efficient way to assess credit risk by processing large datasets quickly and accurately, making your financial decisions smarter and more effective.
How Can Financial Ratios Be Utilized To Estimate Default Probability?
Financial ratios are essential for estimating the probability of default.
Here’s how you can use financial ratios to estimate default probability:
- Analyzing Financial Health: You can assess a company’s liquidity, leverage, and overall financial stability using ratios like current ratio, debt-to-equity ratio, and profitability ratios. Strong indicators typically suggest a lower probability of default.
- Predictive Modeling: By incorporating financial ratios into statistical models like logistic regression, you can predict default probability. These models analyze the relationship between financial ratios and historical default data to estimate future risks.
- Credit Risk Models: Banks and financial institutions use financial ratios in their internal rating systems and credit risk models. These models, compliant with Basel and other regulations, help you assess a borrower’s repayment capacity and estimate expected credit losses.
- Sector-Specific Analysis: Tailoring financial ratios to specific industries, like prioritizing inventory turnover in manufacturing or profit margins in services, increases the accuracy of your default probability estimations.
As a final point, by understanding and applying these financial ratios, you can more accurately gauge the likelihood of a borrower defaulting on their obligations, making more informed decisions.
What Are The Differences Between Statistical And Structural Credit Risk Models?
Statistical and structural credit risk models have distinct differences that impact how you assess credit risk.
Statistical models rely on historical data to estimate the probability of default (PD) and potential losses. These models differentiate between defaulters and non-defaulters using observable factors like financial ratios and credit scores. Examples include logistic regression and linear discriminant analysis.
Structural models are based on corporate finance theory and examine a firm’s assets and liabilities to determine default probability. In these models, default occurs when a firm’s asset value drops below its liabilities. The Merton model, which uses option pricing theory to estimate credit risk, is a well-known example.
Key Differences:
- Approach: Statistical models use historical data and statistical methods without assuming specific theoretical causal relationships. Structural models leverage financial theories and formal relationships between variables.
- Predictors: Statistical models incorporate financial ratios, credit scores, and macroeconomic indicators. Structural models focus on the firm’s asset values, liabilities, and market data.
To sum up, understanding the differences between statistical and structural credit risk models helps you get a comprehensive view of credit risk by considering both empirical data and theoretical frameworks.